
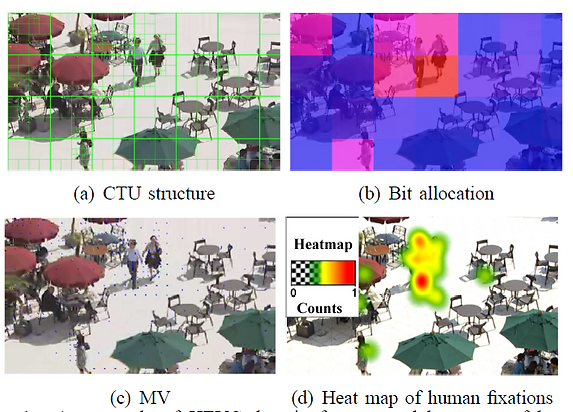
Learning to Detect Video Saliency with HEVC Features
-
Abstract
-
Motivation
Saliency detection has been widely studied to predict human fixations, with various applications in computer vision and image processing. For saliency detection, we argue that the state-of-the-art high efficiency video coding (HEVC) standard can be used to generate the useful features in compressed domain. Therefore, this paper proposes to learn the video saliency model, with regard to HEVC features. First, we establish an eye tracking database for video saliency detectio. Through the statistical analysis on our eye tracking database, we find out that human fixations tend to fall into the regions with large-valued HEVC features on splitting depth, bit allocation, and motion vector (MV). In addition, three observations are obtained with the further analysis on our eye tracking database. Accordingly, several features in HEVC domain are proposed on the basis of splitting depth, bit allocation, and MV. Next, a kind of support vector machine (SVM) is learned to integrate those HEVC features together, for video saliency detection. Since almost all video data are stored in the compressed form, our method is able to avoid both the computational cost on decoding and the storage cost on raw data. More importantly, experimental results show that the proposed method is superior to other state-of-the-art saliency detection methods, either in compressed or uncompressed domain.
High efficiency video coding (HEVC) was formally approved as the state-of-the-art video coding standard in April 2013. It achieves double coding efficiency improvement over the preceding H.264/AVC standard. Interestingly, we found out that the state-of-the-art HEVC encoder can be explored as a feature extractor to efficiently predict video saliency. As shown in figure, the HEVC domain features on splitting depth, bit allocation and motion vector (MV) for each coding tree unit (CTU), are highly correlated with the human fixations.
-
Method
Three HEVC features, i.e., splitting depth, bit allocation, and MV, are effective in predicting video saliency. Therefore, they are worked out as the basic features of video saliency detection. Besides, based on the three basic HEVC features, the features on temporal and spatial difference are also developed, followed by a proposed camera motion removing algorithm. As shown in this figure, given the HEVC bitstreams, all HEVC features need to be extracted and calculated. Then, the saliency map of each single video frame is yielded by combining the HEVC features with C-support vector classification (C-SVC), which is a kind of non-linear SVM classifer. Here, the C-SVC classifer is learned from the ground-truth human fixations of training videos. At last, a simple forward smoothing filter is applied to the yielded saliency maps across video frames, outputting the final video saliency maps.
An example of HEVC domain features and heat map of human fixations for one video frame.

Framework of our HEVC feature extractor for video saliency detection.

Framework of our learning based method for video saliency detection with HEVC features.
-
Results
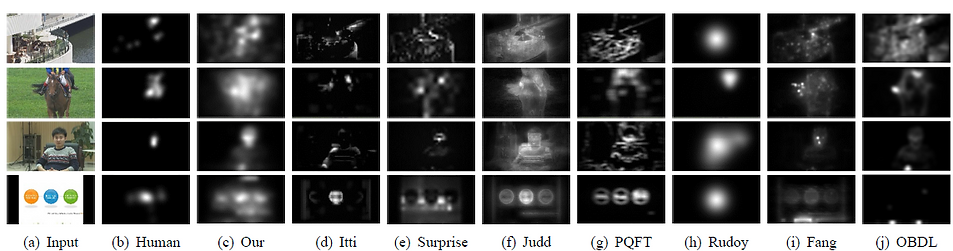
Saliency maps yielded by our and other 7 methods as well the ground-truth human fixations.
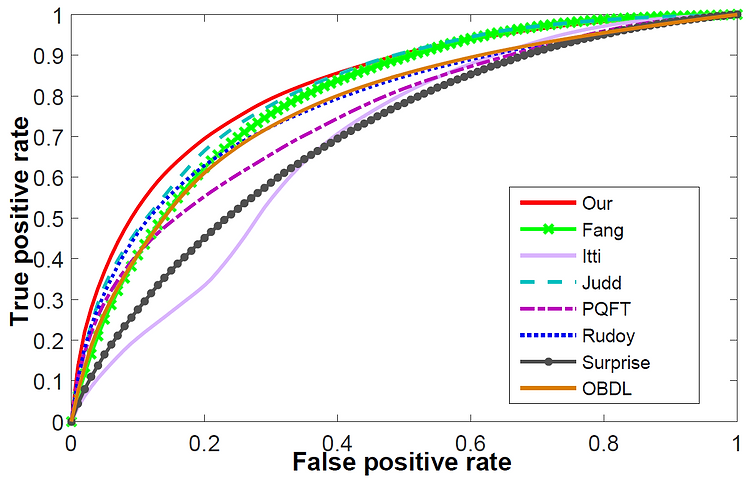
The ROC curves of our and other saliency detection methods.
-
Publication
​
-
Mai Xu, Lai Jiang, Xiaoyan Sun, Zhaoting Ye, Zulin Wang. Learning to Detect Video Saliency with HEVC Features. IEEE Transactions on Image Processing 2017.
The averaged accuracy of saliency detection SFU and DIEM.
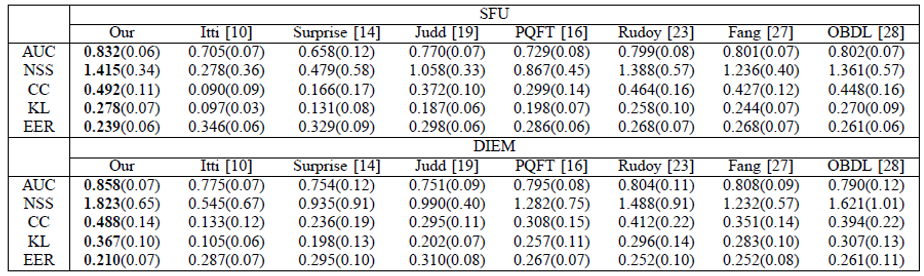
The averaged accuracy of saliency detection on test videos of cross-validation over our database.
