
-
Videos
We conducted the eye tracking experiment to obtain fixations on viewing videos of the latest test sets. Here, all 33 raw videos from the standard test sets, which have been commonly utilized for evaluating HEVC performance, were included in our eye tracking experiment. We further conducted the extra experiment to obtain the eye tracking data on watching all videos of our database compressed by HEVC at different quality. Through the data analysis, we found that visual attention is almost unchanged when videos are compressed at high or medium quality (more than 30 dB). Compared with the conventional databases (e.g., SFU and DIEM ), the utilization of these videos benefits from the state-of-the-art test sets in providing videos with diverse resolutions and content. For the resolution, the videos vary from 1080p to 240p. For the content, the videos include sport events, surveillance, video conferencing, video games, videos with the subscript, etc.
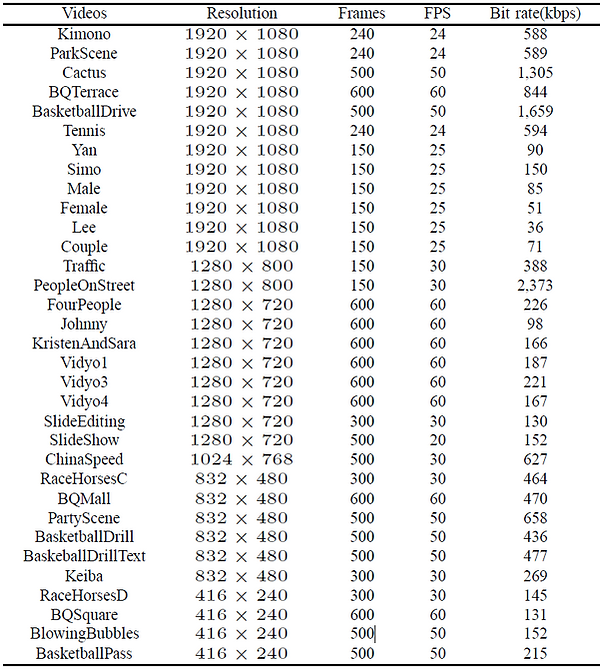
The detailed information for all 33 videos.
-
Procedure
In our experiment, the videos were displayed in a random manner at their default frame rates. Besides, a blank period of 5 seconds was inserted between two consecutive videos, so that the subjects can have a proper rest time to avoid eye fatigue. There were a total of 32 subjects (18 male and 14 female, aging from 19 to 60) involved in our eye tracking experiment. All subjects have either corrected or uncorrected normal eyesight. Note that only two subjects were experts, who are working in the research field of saliency detection. The other 30 subjects did not have any research background in video saliency detection, and they were also native to the purpose of our eye tracking experiment. The eye fixations of all 32 subjects over each video frame were recorded by a Tobii TX300 eye tracker at a sample rate of 300 Hz. All subjects were seated on an adjustable chair at a distance of around 60 cm from the screen of the eye tracker, ensuring that their horizontal sight is in the center of the screen. Before the experiment, subjects were instructed to perform the 9-point calibration for the eye tracker. Then, all subjects were asked to free-view each video. After the experiment, 392,163 fixations over 13,020 frames of 33 videos were collected.
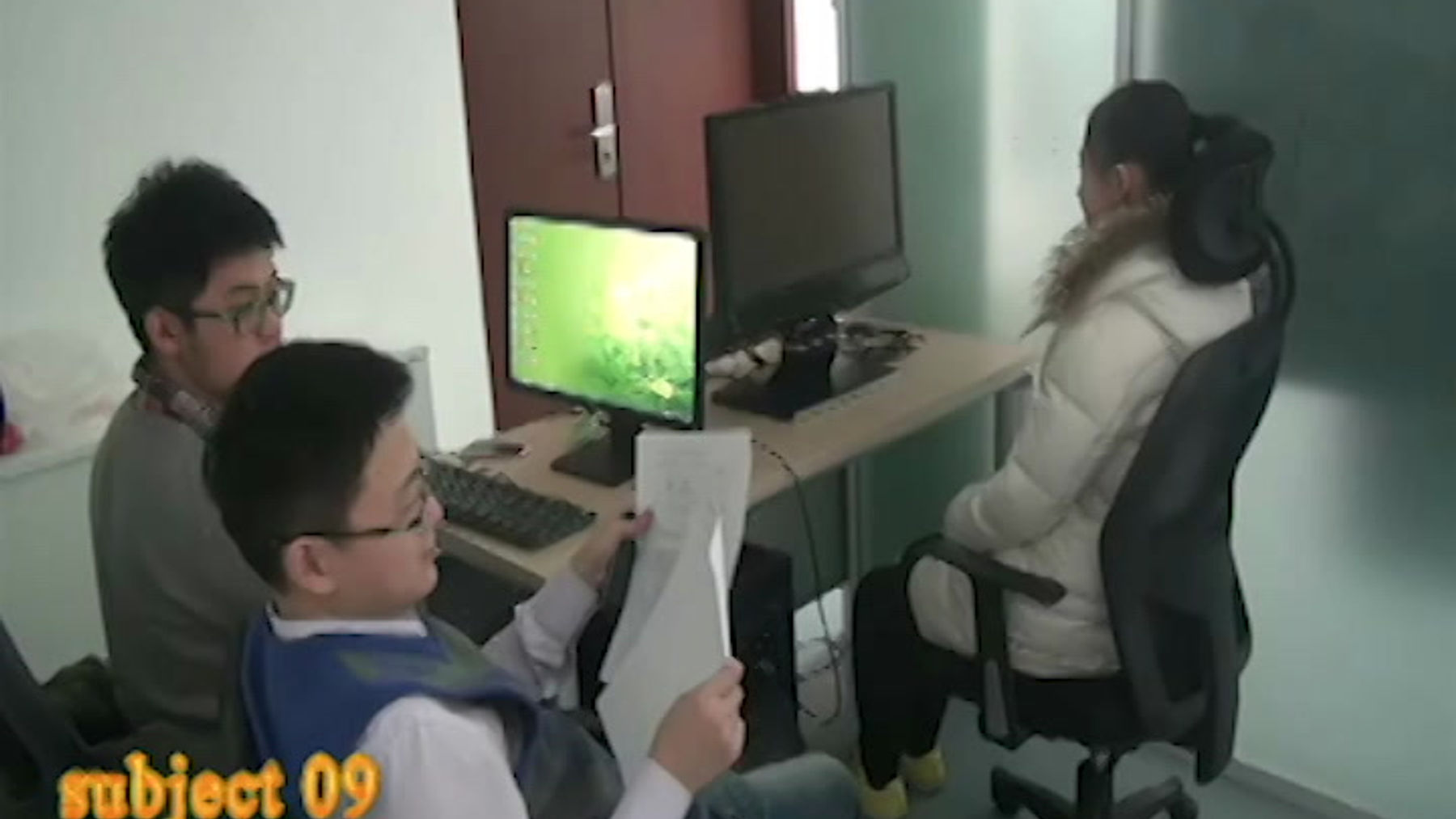
Eye-tracking
The procedure of the eye-tracking experiment.
-
Observations
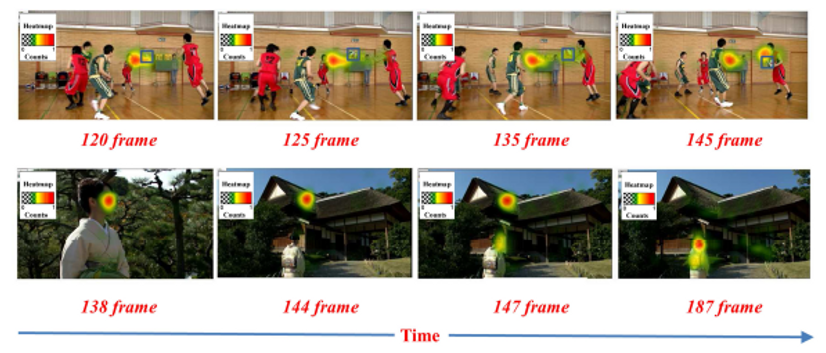
Illustration of Observation 1.
1. Human fixations lag behind the moving or new objects in a video by some microseconds.
In the figure below , we show the frames of two videos with the corresponding heat maps of human fixations. The first row of this figure reveals that the visual attention falls behind the moving object, as the fixations trail the moving basketball. Besides, the second row of figure illustrates that the human fixations lag behind the new appearing objects by a few frames. It is because the human fixations still stay in the location of the salient region in previous frames, even when the scene has been changed.
2. Human fixations tend to be attracted by the new objects appearing in a video.
It is intuitive that visual attention is probably to be attracted by the objects newly emerging in a video. It is thus worth analyzing the influence of the object emergence on human visual attention. In the figure below, a person appears in the door from the 553-th frame of the first video, and that a person riding bicycle arises from the 64-th frame of the second video. The heat maps show that once a new object appears in the video, it probably attracts a huge amount of visual attention.
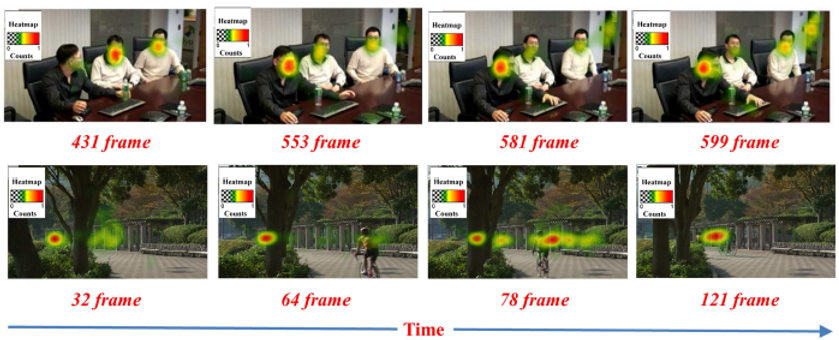
Illustration of Observation 2.
3. The object, which moves in the opposite direction of the surrounding objects, is possible to receive extensive fixations.
Previous work has verified that the human fixations on still images are influenced by the center-surround features of color and intensity. Actually, the center-surround feature of motions also has an important effect on attracting visual attention. As seen from figure below, the old man with a trolley moves in the opposite direction of the surrounding crowd, and he attracts the majority of visual attention. Therefore, this suggests that the object moving in the opposite direction to its surround (i.e., it is with large center-surround motion) may receive extensive fixations.
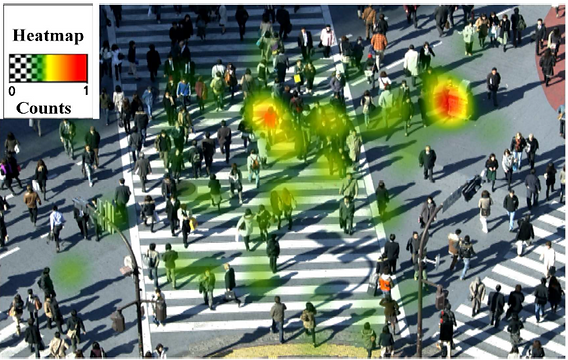
Illustration of Observation 3.
-
Demos
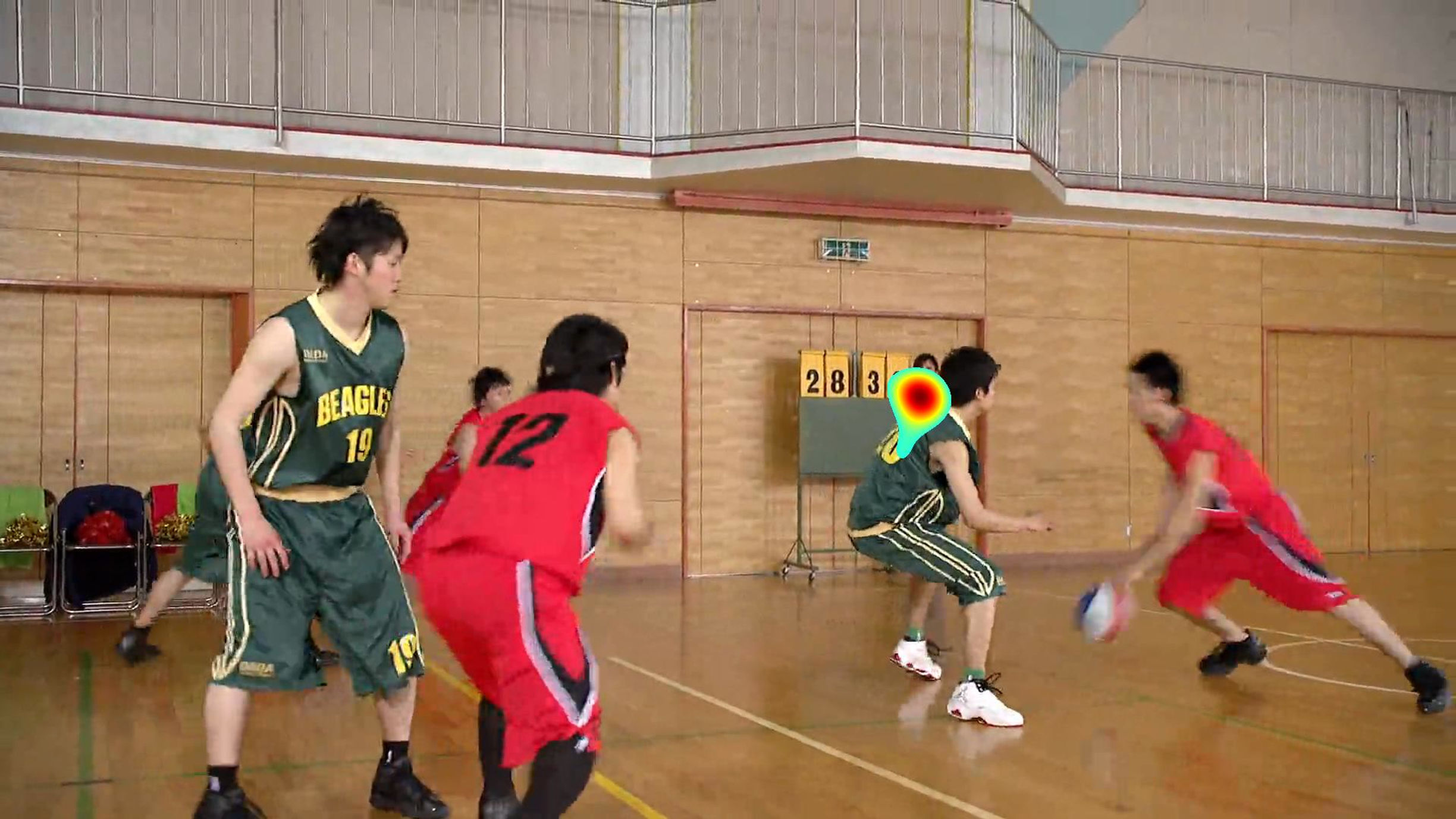
Raw video dataset
-
Reference
-
Mai Xu, Lai Jiang, Xiaoyan Sun, Zhaoting Ye, Zulin Wang. Learning to Detect Video Saliency with HEVC Features. IEEE Transactions on Image Processing 2017.